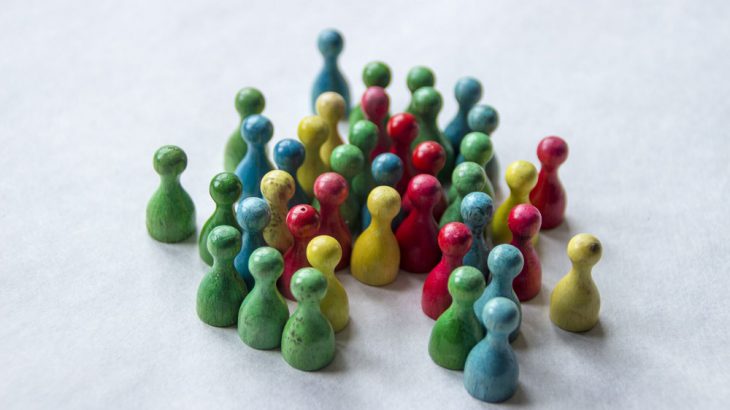
Estimating Default Risk in Fund Structures
We recently hosted a series of Knowledge Management sessions titled “Spark” on an array of topics. Over the coming days we will be sharing notes and proceeds from these sessions. We begin with proceeds from Ravi Saraogi’s session on “Estimating Default Risk in Fund Structures” where he talked about why such estimation is a challenging task and how this process has evolved over the years and the current methodologies for assessing such risks.
By Ravi Saraogi
Estimating default risk in Fund structures can be challenging. One way to assess the credit rating of a Fund structure or a Collateralized Debt Obligation (CDO) is to use the weighted-average rating (WAR) of the underlying asset pool. However, such a measure does not sufficiently describe credit quality. Two portfolios with the same WAR could have very different risk and return characteristics. A portfolio with only BB rated securities compared to a portfolio with some AA and many CCC rated securities can have the same WAR, but will have very different volatility indicators.
Several techniques have been used to assess credit risk in Fund structures and CDOs. Unlike a bond, whose stand-alone credit rating is sufficient to communicate riskiness, the credit quality of a collection of bonds under a Fund structure cannot be easily imputed. Below, we describe the prominent rating methodologies for such structures.
Moody’s Binomial Expansion Technique
Moody’s Binomial Expansion Technique (BET) is one of the earliest known techniques of credit rating. Introduced in 1996, it continues to be used in Fund and Collateralized Debt Obligations (CDOs) analysis alongside other methods. Under this method, a hypothetical portfolio of uncorrelated and homogenous assets is created from the original asset pool. The number of assets in this hypothetical subset portfolio is termed as the ‘diversity score’ (DS). Given homogeneity and the non-correlation in the hypothetical portfolio, DS+1 default situations are captured and the probability of each default scenario occurring is derived through a binomial formula. The expected loss (EL) is then calculated as the product of default probability and the loss severity in the event of a default. Based on the DS+1 default simulations, the loss distribution curve is derived which is compared to a target loss distribution to check if the credit enhancement is adequate1.
There are a number of limitations associated with the BET model, the most significant being its restrictive assumptions. The assumption of zero correlation in the constructed hypothetical portfolio means that the EL will be accurately calculated only when there is, in fact, no correlation in the asset pool. This means BET fails the accuracy test due to the presence of correlation in the larger asset pool. Indeed, empirical tests show that ‘BET underestimates EL when the collateral assets are correlated, with the degree of underestimation rising in the subordination level’2. Another drawback of BET is that it uses discrete probability distribution as compared to continuous distribution in the Monte Carlo (MC) simulation approach.
Standard & Poor’s Monte Carlo Simulation Technique
The difficulty of deriving a credit rating for a Fund structure or a CDO from the credit rating of the underlying portfolio, either in terms of the applicability of WAR or BET or mathematical imputations, has led to the widespread adoption of simulation in CDO credit ratings3.
S&Ps rating approach to CDOs can be broken into two steps. In the first step, an expected loss distribution for the underlying assets is estimated. In the second step, cash flow simulations are conducted to check if a particular tranche can withstand the required level of defaults for a given rating.
The expected loss distribution is estimated using MC simulations based on the historically observed Cumulative Default Rate (CDRs) for the underlying rated assets and correlation assumptions. Post the derivation of the expected loss distribution, two statistics are computed by S&P – the ‘scenario default rate’ (SDR) and the ‘breakeven default rate’ (BEDR). The SDR is defined as the extent of default in the underlying asset pool that a tranche must be able to withstand to secure the rating assigned to it, while the BEDR indicates the actual level of default in the underlying asset pool which a tranche sustains based on cash flow simulations4.
Next, the cash flow simulations are run in the underwriter’s cash flow model. If the BEDR is more than (or equal) to the SDR, the credit enhancement for that tranche is higher (or equal) than what is required for assigning that rating to the pool.
Imputing Credit Rating Through Default Instances
An intuitive way to assess credit risk in Fund structures is to use MC simulation to compute the number of default instances and compare the same to historical CDRs. This can be done by taking the cash flow outputs from the underwriter’s model and running it past the Fund or CDO payment waterfall. For each such simulation, a counter can be built to record if cash flows were sufficient to meet payment commitments or not. At the end of the consolidated simulation, the default rate can be mapped to the historical corporate bond default rate matrix for imputing the credit rating.
The above provides a brief introduction on the different approaches for estimating default risk in Fund structures and the same has been discussed in detail in the presentation. These methodologies have witnessed increased scrutiny post the US subprime crisis when several highly rated Fund and CDO structures went bust5,6. The crisis highlighted the need to better understand risk when assets are pooled under different investment structures; distinct from evaluating risk in a single financial security.
View the video from the session below:
- Garcia, J., et al. (2004), On Rating Cash Flow CDO’s using BET technique. http://www.geocities.ws/joaogarcia18/DexiaCreditMethodology/CDOBETPaper60Web.pdf
- Fender, I., Kiff, J., 2004. CDO rating methodology: Some thoughts on model risk and its implications. Bank of International Settlements Working Papers No.163
- Myers, S., 1976. Postscript: Using Simulation for Risk Analysis- Modern Developments in Financial Management. Praeger Publications. pp. 457–463.
- Standard & Poor’s. 2002. Global Cash Flow and Synthetic CDO Criteria. S&P Structured Finance.
- Benmelech, E., Dlugosz, J., 2009. The alchemy of CDO credit ratings. Journal of Monetary Economics.56, 617-634.
- Griffen, J.M., Tang, D.Y., 2011. Did Subjectivity Play a Role in CDO Credit Ratings? Journal of Finance. Volume 67, Issue 4, pages 1293–1328.